Rapidly rising autism rates could be due to over diagnosis and poor diagnostic criteria, a provocative new study suggests.
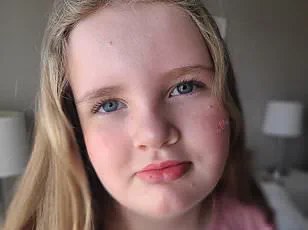
Utilizing an AI algorithm, researchers at the Montreal Neurological Institute-Hospital and Quebec Artificial Intelligence Institute analyzed more than 4,000 clinical reports from children being evaluated for autism in Quebec.
The Diagnostic and Statistical Manual of Mental Disorders, Fifth Edition (DSM-5), serves as the gold standard for diagnosing mental conditions.
It includes criteria such as avoiding eye contact, highly limited interests, repetitive movements, and difficulty forming friendships or engaging in back-and-forth conversations.
The researchers aimed to measure which diagnostic criteria were most often used in autism evaluations.
The study revealed that social-related behaviors like nonverbal communication and forming relationships were not specific indicators of an Autism diagnosis.
These behaviors appeared frequently even among individuals not diagnosed with the condition, indicating a lack of specificity for these traits when diagnosing autism.
In contrast, repetitive movements—also known as ‘stimming’—and hyperfixations showed strong links to an autism diagnosis.
The researchers contend that current diagnostic practices may be over-identifying children with autism based on social-related factors while neglecting behaviors more closely associated with the condition, such as stimming.
They advocate for a streamlined approach to autism evaluations, emphasizing the importance of focusing on non-social behavioral indicators and utilizing AI programs for language evaluation.
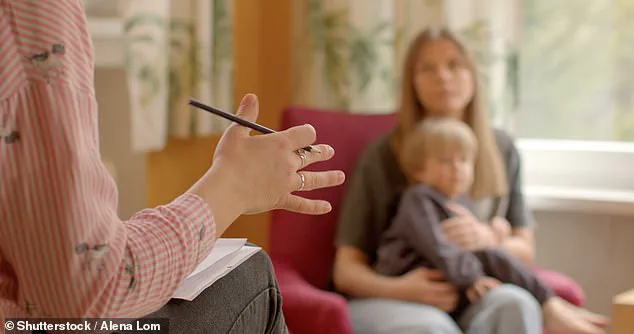
According to Dr.
Danilo Bzdok, a neuroscientist involved in the research, advancements in large language model technologies could play a crucial role in re-evaluating diagnostic criteria for autism in the future.
This innovative approach promises to enhance both the effectiveness and efficiency of diagnosing autism, potentially leading to quicker access to appropriate therapies and treatments.
While there is currently no cure for autism, various therapeutic interventions like Applied Behavioral Analysis (ABA) and certain medications can help improve behaviors associated with the condition.
The findings from this study underscore the need for a more refined diagnostic process that accurately captures the core characteristics of autism without conflating broader social behavior patterns.
Researchers recently employed a sophisticated large language model—a type of artificial intelligence designed to process and understand human language—to analyze diagnostic reports for signs predictive of an autism diagnosis.
The study included data from 1,080 participants, among whom 429 were officially diagnosed with autism by medical professionals.
These children averaged seven years old at the time of assessment.
The researchers fed the Diagnostic and Statistical Manual of Mental Disorders, Fifth Edition (DSM-5)’s seven criteria for diagnosing autism into their AI model.
The DSM-5 outlines behaviors such as difficulty sharing interests or having conversations with others, challenges in non-verbal communication like making eye contact, difficulties maintaining relationships with peers, repetitive movements and mimicking, rigid adherence to routines or resistance to change, highly restricted interests, and increased sensitivity to sensory stimuli.
The AI model identified that participants diagnosed with autism were most likely to exhibit the DSM-5’s criteria related to non-social behaviors.
These include repetitive actions, echolalia (repeating sounds or words), narrow areas of interest, and heightened sensitivity to sensory input.
Based on these findings, the researchers suggested a more focused approach in evaluating children for autism by prioritizing non-social characteristics over social ones during diagnosis.
The experts also advocated for a re-evaluation of diagnostic criteria to enhance accuracy and prevent overdiagnosis.
However, they noted several limitations, including insufficient data on older children whose symptoms might differ significantly from those of younger children.
This study arrives at a time when the United States is witnessing a surge in autism diagnoses.
According to recent CDC data, one in 36 American children now has an autism spectrum disorder diagnosis—approximately two million individuals nationwide.
This represents a substantial increase since the early 2000s when the rate was closer to seven per thousand.
Most individuals with autism are diagnosed by age five, though some can be identified as young as two years old.
A study published last year in JAMA Network Open revealed that between 2011 and 2022, there was a dramatic rise in diagnoses among children aged five to eight, increasing from two per thousand to six per thousand—a 175 percent increase over the decade.
However, the most significant jump occurred among young adults aged 26 to 34, with a staggering 450 percent increase, suggesting that many of these individuals had delayed diagnoses earlier in life.
The surge in autism diagnoses is attributed not only to better detection by medical professionals but also to potential environmental factors.
While experts acknowledge the role of improved diagnostic practices, they caution that the exact causes of autism remain poorly understood and likely involve multiple contributing factors rather than a single definitive cause.
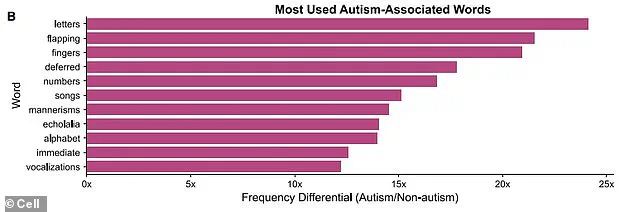
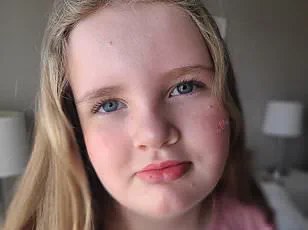
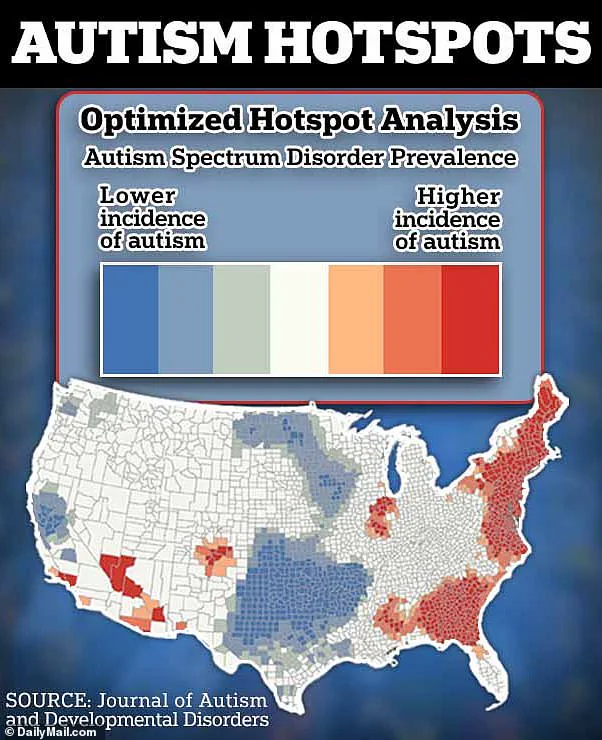
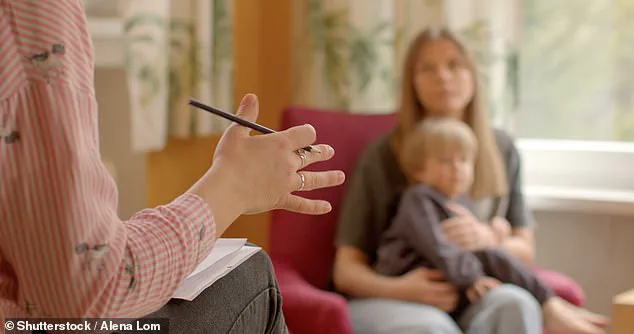